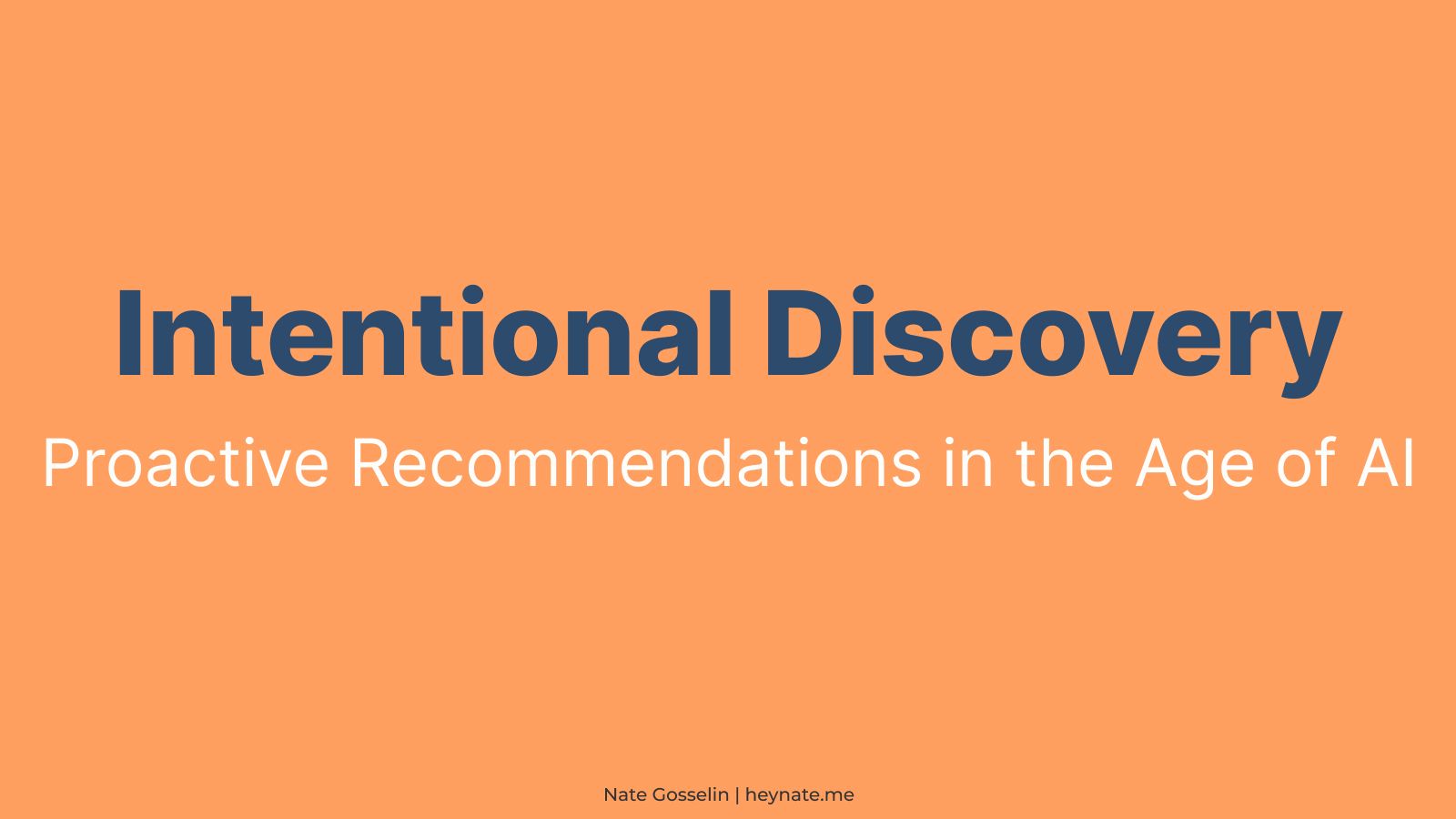
In my piece Planting Knowledge Trees, I touched upon how AI can be a game-changer in exploring new territories, especially in fields that are personally uncharted. Today, I want to delve deeper into a concept I’ve been mulling over: proactive recommendation. I’m excited by this concept because it is a tangible strategy with the potential to enhance the way we discover new music, books, movies, and beyond.
Before we leap into the depths, let’s clarify what I mean by proactive recommendation. It’s a method of discovery that enables individuals to actively seek out new interests and knowledge, guided by AI. Unlike the traditional passive systems where algorithms dictate your next find, proactive recommendation puts you in the driver’s seat, with AI illuminating the path ahead. This approach fosters a more personalized and engaged exploration of content.
Lately, Spotify has been at the forefront of personalized music discovery. And to be clear, they’re doing it an elite level: the auto-curated Spotify features like the Daylist and the array of Daily Mixes have become my go-to habit for music consumption. While I’m not privy to the code, the essential approach of these playlists is to recommend music based on your listening history. The product analyzes my listening habits, identifies patterns, and curates playlists with a mix of old and new songs that align with my tastes. Recently, what’s been cool (and revealing) about Spotify’s Daylist is how they surface the relevant music categories contained in the playlist. In turn, you’re able to click into the category and receive a whole new playlist of music in that vein.
This system builds upon the extensive recommendation work at Pandora, whose Music Genome Project famously enlisted an army of musicologists to classify songs based on 450 unique attributes. Pandora used this classification system to essentially orchestra a sophisticated process-of-elimination with each station, using your up/down votes to slowly zero in on the collection of attributes that songs needed to have. Taken together, these two products illuminate a template of sorts for recommendations — leveraging past usage to suggest new content.
While this template is powerful, there are some inherent limitations in how they use listening history to build recommendations. For one, it’s entirely passive; you’re at the mercy of algorithms to serendipitously stumble upon new tunes. The passivity also forces the product to only gradually expand your exposure to new music — this can sometimes lead to being stuck in a repetitive loop. On Spotify, for example, there’s a pool of about 100-200 songs that seem to show up on almost all of my playlists.
What I think is interesting about both the Spotify and Pandora models is how they leverage domain-specific taxonomies to power their recommendations. Whatever their data curation methods, they’ve built up an impressive library of specific musical categories that they can use to precisely tailor music for the listener. But imagine flipping the script and putting users in control of selecting these categories themselves. Traditionally, this would require users to be domain experts, but with AI, that barrier is removed.
Here’s where AI steps in to shift the paradigm. By exposing the underlying taxonomy, AI can give users the language and framework to embark on their own journey of discovery. For instance, when I query ChatGPT about the characteristics of a specific musical artist, it not only identifies those characteristics but also enables me to explore similar genres or artists with ease. This dialogue with AI opens up a new world of discovery, allowing me, and any user for that matter, to direct their exploration with precision:
What’s powerful about this approach is how it can be expanded to other domains, like book recommendations or food recommendations.
Proactive recommendations potentially signify a sea change in how we engage with content, moving beyond the realm of music and movies to revolutionize the discovery process across all types of media. This approach places users in control, equipping them with the necessary tools to actively navigate the expansive content landscape. It aims to disrupt the usual cycle of passive content consumption, fostering a more dynamic and fulfilling interaction with media. For product teams dedicated to discovery, the adoption of AI-driven domain taxonomies presents a significant opportunity to enhance the user experience, offering a more captivating, personalized, and gratifying exploration of content. This innovation seeks not just to satisfy users but to fundamentally alter the dynamics of discovery, encouraging a proactive quest for new interests.
However, the transition towards proactive recommendations comes with its set of challenges, including ethical considerations around personalization, privacy, and the compensation of creators who provide the training data. As this field evolves, the role of AI advancements will be crucial, necessitating continuous adjustments and improvements. The potential of leveraging AI to create domain taxonomies and transform discovery platforms is immense, marking a departure from passive consumption towards a more intentional, user-directed exploration of content. Such an evolution promises to not only enrich personal experiences but also to forge a future where discovery is limitless, tailored, and profoundly interactive. This is the potential of proactive recommendation, a concept ripe with possibilities for transforming how we explore the world around us.
Footnotes
Enjoyed this post? Get more like it in your inbox.